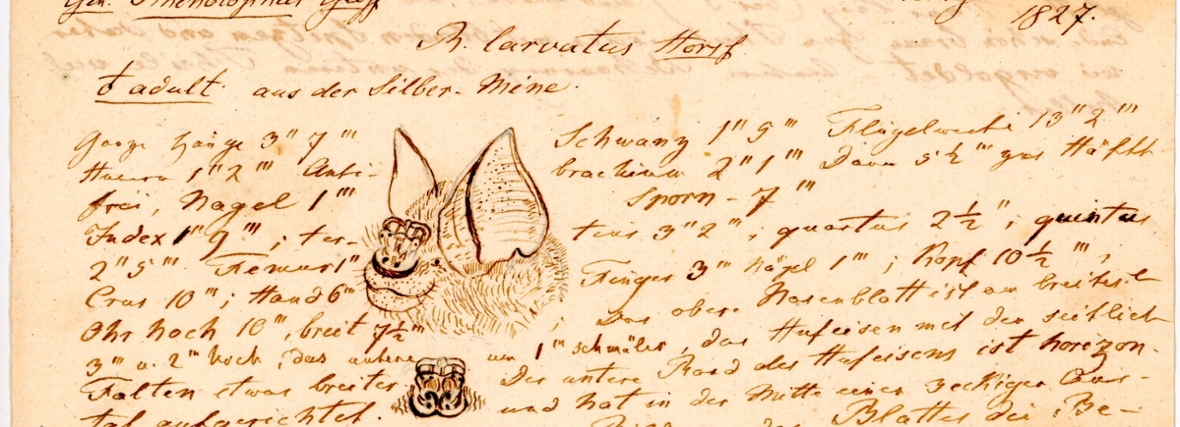
Integrating data to learn more
Tremendous amounts of data are generated in scientific research each day. Most of this data has more potential than we are using now, says Katy Wolstencroft, assistant professor in bioinformatics and computer science. We just need to integrate and manage it better.
What is data integration and why is it so important?
‘There’s an awful lot of data available, and to really improve our knowledge we should be able to use all of it. Humans can only do that to a certain extent: we can read papers and build on the conclusions in those. But that’s only a small piece of the puzzle. In order to make use of all the data that has been generated before, we need computers to help us. We could gain a great deal of knowledge by combining existing datasets. But for a variety of technical reasons, it’s not so easy to stitch together data from different types of research. Data integration is needed to make this possible.’
Could you give an example of how this can be done?

‘Making data FAIR is an important step. FAIR stands for Findable, Accessible, Interoperable and Reusable. It involves publishing datasets in a format that can be understood by humans and computers. In order to do that, we have to be very systematic in how we classify and describe things. Once data is FAIR, we can use computers to identify new connections and discover patterns in it. This way, data can answer many different questions, not just the specific question that it was generated for in the first place.’
How can we make this happen?
‘One of the main projects I’m involved in, FAIRDOM, is a good example. It’s a platform where researchers from the field of systems biology share their data and mathematical models – with the world, or just with other researchers. The platform helps researchers structure and annotate their research results. We help them with their data management and provide them with tools to make their data FAIR. For instance, we’ve developed a tool called RightField, which embeds semantic annotation into spreadsheets.’
Do these researchers have to adopt a whole new way of working with data?
‘No, we have to be realistic about how much time people can put into this. For most biologists, data management is not one of their main interests, so we have to make sure it takes as little time as possible and we have to make sure it is straight-forward to standardise and annotate data. That’s why we developed all these tools that require only a little more effort, instead of telling people they should completely change the way that they work.’
Recently, some of the most important scientific journals called for researchers to adopt the principles of FAIR. It seems like FAIR, which originated here in Leiden, is quickly going global.
‘Yes, I’m very pleased with the way it is spreading, from the European Commission all the way down, through to institutes and individual researchers. Some funding councils now require researchers to include FAIR in their project proposals, which is great. I hope that in the future, allowing your data to become part of the pool of our collective knowledge will be part of every researchers’ workflow. Then we could learn a lot more, and more quickly.’
You’re also involved in a different project: Making Sense of Illustrated Handwritten Archives. What does that entail?
Naturalis, and many other museums like it, have rooms full of archive material: collections of field notes and specimens from the last few hundred years. The aim of the Making Sense project is to develop methods to automatically extract the research data contained in these archives. So this project, too, is about data integration. For the Making Sense project, we have material about the natural biodiversity of Indonesia in the early 1800s. A lot of this data is digitized, so scans of hundreds of pages have been made available, but the material is in multiple different languages, in hard-to-read handwriting. This makes existing methods for automatically disclosing the content unsuitable. What we do is develop methods to make the data more accessible to researchers, for comparison and for searching.’
How do you go about that?
‘We collaborate with a research group at Groningen University, which has developed an adaptive learning system for handwriting recognition. Here in Leiden, we built a semantic model to describe what we’re trying to find out from each handwritten record, and how that links to archive illustrations and specimens. Instead of transcribing every page, we look for the most important concepts. Like the species’ name and attributes, where it was found, who found it, how many of it, etcetera. We use image recognition and layout analysis to help us learn where these things appear in the field book pages.’
You started out in biochemistry. How did you end up in the field of computer science?
‘That was slightly accidental, actually. When I finished my undergraduate degree, I wanted to become a forensic scientist. But I wasn’t really sure, and in the mean time I found a job opening as a research assistant in bioinformatics. So, I thought I’d gain a bit of experience in that. At the time, bioinformatics was just beginning to take off. The amount of available data was exploding due to advances in genome sequencing. A whole new field opened up, it was an exciting time. So even though I ended up in this field by accident, I realized quickly that I wanted to stay in it. And I did.’
Katy Wolstencroft is an Assistant Professor at the Leiden Institute of Advanced Computer Science (LIACS), where she does research in semantic data integration, primarily in systems biology. She studied biochemistry at the University of Leeds (undergraduate) and bioinformatics (MSc and PhD) at the University of Manchester. Wolstencroft was a Post-Doc and Research Fellow at the School of Computer Science, University of Manchester and was also a visiting Research Fellow at the Vrije Universiteit, Amsterdam.
This article is part of a series of interviews with researchers from the Leiden Centre of Data Science (LCDS). LCDS is a network of researchers from different scientific disciplines, who use innovative methods to deal with large amounts of data. Collaboration between these researchers leads to new solutions to problems in science and society.